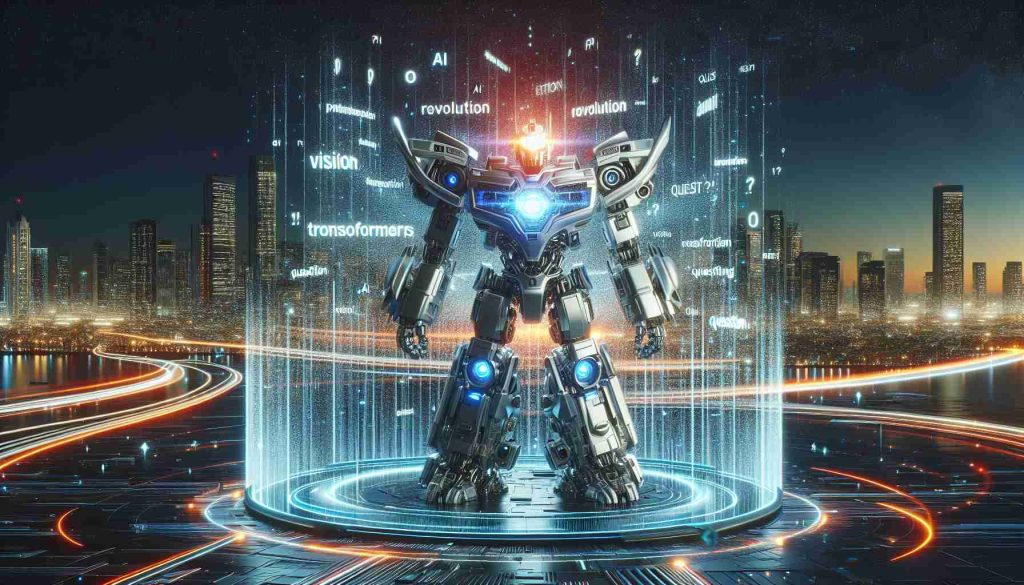
AI Revolution: Vision Transformers Get Question-Savvy
In the fast-evolving landscape of artificial intelligence, the integration of vision transformers with question-awareness represents a groundbreaking leap in multimodal reasoning. The newly developed “Question Aware Vision Transformer” (QAVT) is designed to enhance AI systems’ ability to interpret and correlate visual and