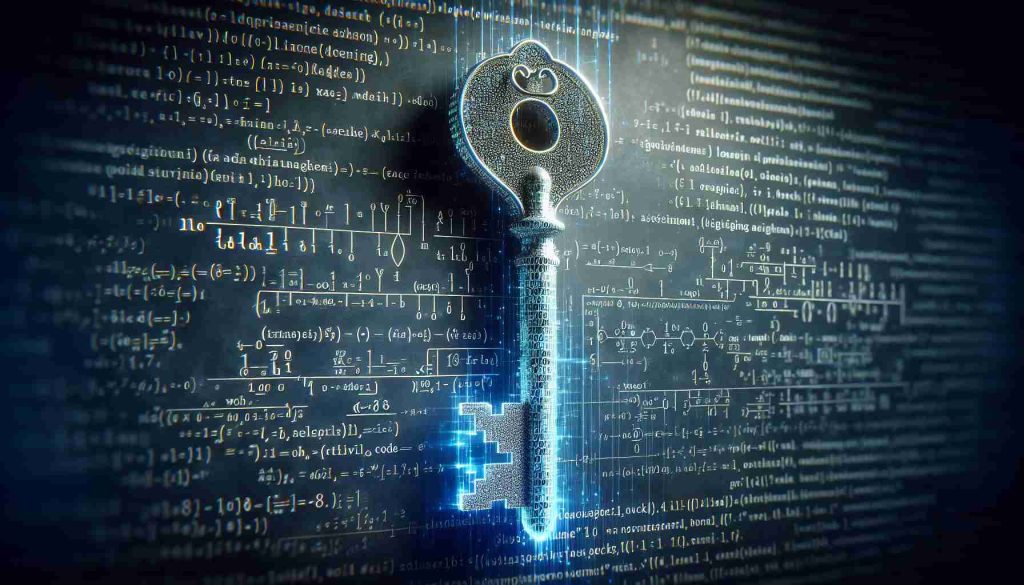
Unlock the Secrets of Machine Learning with This Week 8 Assignment Trick
In the world of data science and artificial intelligence, few things are as fascinating and challenging as machine learning. By the eighth week of a typical machine learning course, students are usually diving into complex topics that push their understanding to new