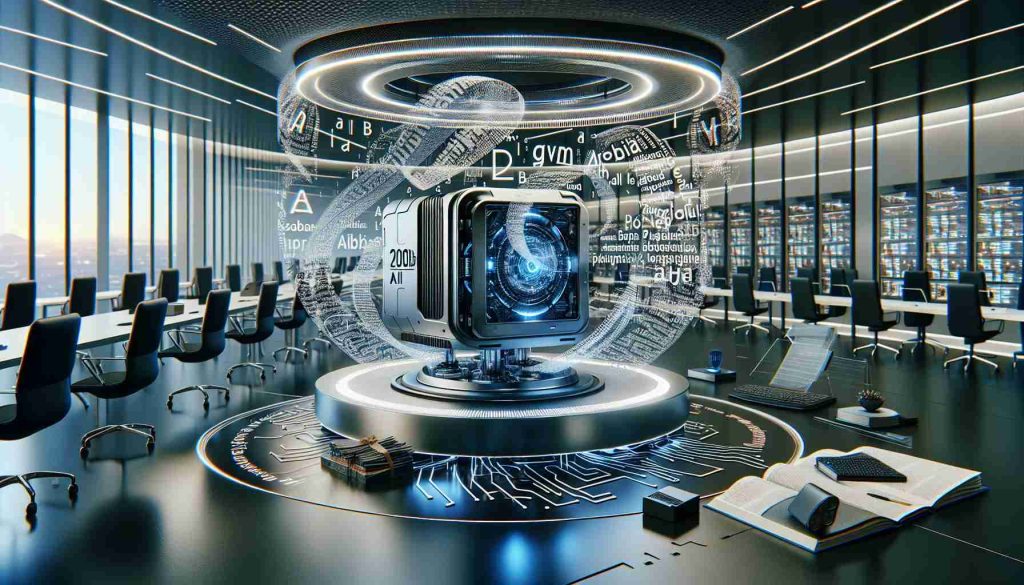
Alphabet’s 200B AI Revolutionizes Language and Challenges the Status Quo
Alphabet unveils the “200B AI” language model with 200 billion parameters, revolutionizing communication and machine learning. It excels in generating human-like text in various languages and dialects, impacting education and global business sectors. The model promises personalized learning and improved translation services,