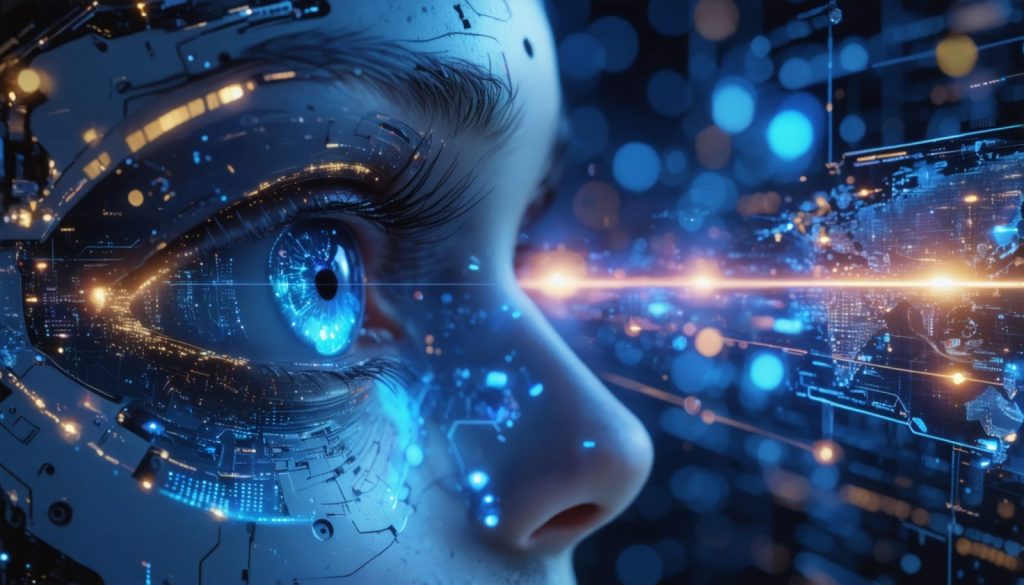
The Future of AI: Geoffrey Hinton’s New Vision Unveiled
Self-supervised learning allows AI to learn from unlabeled data, similar to human learning from the environment. Hinton aims to reduce AI’s dependency on large amounts of annotated data, enhancing efficiency and contextual understanding. He advocates for a balance of cautious optimism in